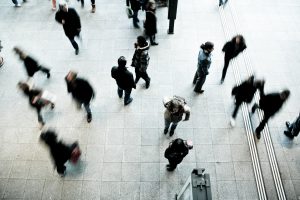
The Department of Sociology is pleased to invite students and colleagues for a seminar hosted by
Øyvind Stiansen
University in Oslo
Causal inference using observational data
The seminar will take place on
March 10th and 12th (Tuesday and Thursday from 13:20 to 16:35
in
Celetná 20, room 207
Social scientists are often interested in estimating causal effects. Did the introduction of a policy, X, produce increased levels of a desirable outcome, Y? Identifying such causal effects requires researcher to identify credible counterfactuals. What levels of Y would have been produced in the absence of X, but keeping everything else the same? Outside the context of a randomized trials, such counterfactuals can be very difficult to establish as unobserved variables are likely to explain both the introduction of X and varying levels of Y. For social scientists relying on observational data, credible causal inference can therefore be hard to achieve.
This course will introduce students to the potential outcomes framework as a general approach to causal inference. Grounded in the potential outcomes framework, the course will cover a number of research designs that may allow for credible causal inference, including conditioning on known confounders, difference-in-differences designs, and instrumental variables. The course will emphasize the assumptions that need to be met for these designs to allow for credible inference and illustrate how they can be applied to social science data.
The Tuesday seminar will offer an introduction to the potential outcomes framework and consider how matching and regression can be used for causal inference under the assumption of selection only observables. The Thursday seminar will consider research designs that may allow for causal inference even in the presence of unobserved confounders, such as difference-in-differences and instrumental variables.
Tha capacity of the seminar is limited. Please, register here: